
Blog
Industry
8 min read
22 Mar 2023
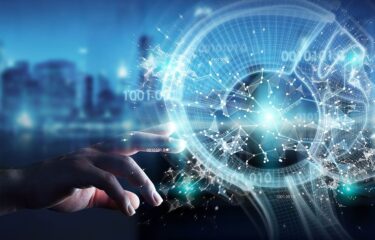
Blog
Industry
8 min read
22 Mar 2023
When embarking upon any working capital optimization programme, buyers should know the answers to three key questions:
While the answers to these questions are fundamental to realizing the benefits of supply chain finance (SCF), in reality, few companies have this information available on-demand. Consequently, much of the global working capital opportunity remains untapped with as much as US$14 trillion currently trapped in supply chains around the world – according to research by the Supply Chain Finance Community and Windesheim University.
For buyers looking to unlock this cash and put it to work, there are many different tools and strategies available, from extending payment terms to securing financing for receivables. But in practice, many such initiatives fall short of their targets. Often this is due to poor visibility over the cash deployed across the enterprise, or a lack of internal consensus about which working capital strategies to pursue first. Companies may also lack tools sophisticated enough to adapt in line with evolving business objectives/ market conditions and can struggle to gauge the impact of working capital programmes on their suppliers.
This is already changing. At the cutting edge of development in this area, leading vendors are using technologies such as artificial intelligence (AI). By doing so, they are giving buyers a far more detailed understanding of the potential benefits of enterprise-wide working capital strategies, and of how different techniques can be deployed to achieve the company’s goals. In practice, this could mean predicting which invoices suppliers will choose to accelerate under a range of different conditions. Or it could mean leveraging the power of AI to extend the cash forecasting process all the way down to the purchase order level. As technology continues to evolve, buyers will be able to unlock working capital and deploy cash into their supply chains more effectively than ever before. So where are the most significant areas of opportunity and how can buyers take advantage of these developments?
First and foremost, any successful working capital strategy needs to be based on a consistent set of facts. Historically, treasury and procurement professionals tended to have very different perspectives on the benefits and risks of different working capital initiatives. Exacerbated by key data being trapped in multiple ERP systems within a single organisation, these differences in opinion can make it difficult to get any working capital strategy off the ground.
The use of AI can help overcome these issues. Combining internal data with third party data not only provides visibility into the organisation’s metrics, but also gives the context needed to understand how the company compares to its peers in areas such as DPO.
Further value is added if the technology vendor’s network data is also incorporated into this shared understanding. If vendors are able to draw upon data from the millions of suppliers that interact with their platforms, buyers will be in a much better position to understand the scale of the working capital opportunity available to them, and the potential impact to their supply chain. They will also be better placed to communicate a single set of quantifiable facts across the organisation.
Also significant is the way technology can be used to identify the optimum approach for suppliers. In recent years, the costs involved in machine learning have plummeted, giving rise to a new era of affordable prediction. Where SCF is concerned, one of the most exciting areas of development lies in running predictive scenarios and assessing whether suppliers will choose to accelerate payments under different conditions.
For example, vendors can feed data from the buyer’s ERP system (along with big data from external sources) into their machine learning to produce predictions about suppliers’ behaviours relative to individual invoices. They may also be able to bring in information about suppliers’ cost of capital and industry expectations regarding DSO. Vendors with strong networks may also be able to draw upon insights from those networks about how suppliers react to offers at varying payment terms, sizes, rates and tenors.
Based on this information, vendors can identify the most suitable payment terms for individual suppliers and determine the level of flexibility that buyers may need in managing those terms. This exercise should be conducted in lock-step with AI to determine the most appropriate APR to offer suppliers. For example, a buyer offering a self-funded dynamic discounting programme could find that there is a 20% likelihood that a specific supplier will opt to accelerate payments at 8% APR. At 6% APR, the probability might go up to 25%, but the net yield would be lower. Conversely, at 10% there might be only a 1% chance that the supplier will accept the offer.
Of course, the likelihood of supplier acceptance is only one part of the equation: the platform also needs to provide insights into the yield that the buyer can expect from early payments based on different APRs and the impact these early payment offers will have on their suppliers’ financial health. In this way, buyers can take advantage of prediction and network insights to find the APR that best balances supplier adoption levels against their required rate of return.
The ability to run ‘what if?’ analysis can also provide valuable insights into the scale of a buyer’s working capital opportunities. This includes enabling buyers to see the impact of injecting more cash into their dynamic discounting programmes, as well as spelling out how this would affect their rate of return.
Vendors drawing upon these developments will be better placed to help buyers realize the opportunities available. As above, data-driven insights could indicate that a different APR would provide better results – but equally, they could suggest that more suppliers should be enrolled on the programme, or that invoices should be approved faster to lengthen the acceleration window (the period between approving an invoice and its due date). In many cases, technology will simply illuminate straightforward operational improvements – albeit with higher precision and greater impact.
In other cases, the available data may suggest that a certain number of suppliers are unlikely to accept the buyer’s lowest available dynamic discounting rate. In such cases, there may be opportunities to switch those suppliers from dynamic discounting terms to the company’s SCF programme. In other words, a Fortune 500 company might not accept dynamic discounting at a 9% APR – but it might accept an SCF offer of LIBOR plus 80 basis points.
Whatever the recommendations, the insights and predictions delivered by technology need to be readily actionable if they are to provide real value. Again, technology can support companies in putting their insights into practice. This could involve automating a planned terms extension for small suppliers. It could also mean arming procurement staff with the knowledge of a given sector’s average payment terms or a supplier’s estimated carrying cost with and without early payments, ahead of payment term negotiations.
Another way in which AI can boost SCF is by providing a clearer view over supplier health, and the impact that different actions could have on the company’s supply chain. As such, technology will increasingly enable buyers to track how the changes they make to their supplier payment terms and SCF programmes affect suppliers over time.
This might include looking at areas such as the supplier’s average payment terms in the market. For example, if a supplier’s market average is 84 days, it may be unreasonable to expect that supplier to move to 180 days payment terms – but 90 days may be a realistic goal. Having access to this type of information is highly likely to prove beneficial when negotiating a terms extension.
Likewise, supplier health metrics might consider the buyer’s own payment performance and whether the buyer is consistently paying suppliers on the agreed date, as well as tracking how frequently suppliers are taking advantage of early payments.
Beyond the working capital benefits of AI and machine learning, technology also has considerable potential when it comes to understanding the cash flow implications of an early payment strategy. For example, by combining the entire document stream with predictive analytics, technology can provide more clarity over not just the invoices that are due but also the probability that suppliers will choose to request early payment on specific invoices. This, in turn, leads to greater visibility over future cash flows:
In this way, technology can not only help companies unlock working capital benefits, but also support more effective forecasting and cash planning.
AI has the power to transform the approach and impact all parties involved in SCF. This transformation is already underway. Today many SCF providers are proactively bringing together the key tools of big data, AI, system integration and network insights gleaned from millions of connections on their platforms. In this way the buyers are empowered to address early payments and free up working capital in a far more strategic way than has previously been possible.
It is clear that the insights provided by AI and machine learning need to translate into action in order to realize cash benefits. Unlike one-off working capital initiatives, which become redundant once the goals of the programme have been met, next generation working capital strategies need to adapt in line with the buyer’s evolving needs in order to provide lasting value.
The possibilities are considerable. By supporting intelligent planning, next generation technology will empower treasury and procurement to pivot their strategies as often as needed, based on the current market environment and business goals. This might mean turning off dynamic discounting offers at certain times, or better yet moving suppliers from dynamic discounting to SCF terms in order to continue supporting suppliers working capital needs. Once buyers understand the different levers available to them, and the impact of pulling these levers to varying degrees, they will be able to gain far greater control over their programme.
Likewise, developments in technology will make it easier for companies to gauge the success of their working capital strategies. Increasingly, buyers will be able to assess their progress in meeting their working capital goals, with visibility over how much cash has been unlocked in different regions or even by different members of the procurement team. This will make it easier for companies to change course when needed, while continuing to make micro adjustments along the way. Greater visibility will also enable buyers to assess the impact of their decisions on supplier health over time.
Developments in AI and machine learning are already reshaping working capital, and further benefits will follow. In the next couple of years, cheaper predictive capabilities will increasingly enable buyers to understand the extent of their working capital opportunity and forecast which suppliers will choose to finance their invoices. At the same time, companies will be better positioned to understand the likely impact of different adjustments to their working capital strategies, whilst benefiting from greater visibility over their future cash flows.
In other words, technology will increasingly support buyers by providing detailed and timely answers to the questions that inform their approach to SCF: “How much trapped cash can I unlock from my supply chain? How much cash should I deploy into my supply chain? How will these actions impact my suppliers?”
Article originally published in BCR on 6 December 2019
Expert Advice
Accounts payable automation software enables easier management of invoicing and payment processing through a single digital platform.
Industry
Treasury departments have a broad range of roles, from cash forecasting to working capital management. And just like every other…